Unlocking the Future with Data Annotation in Machine Learning
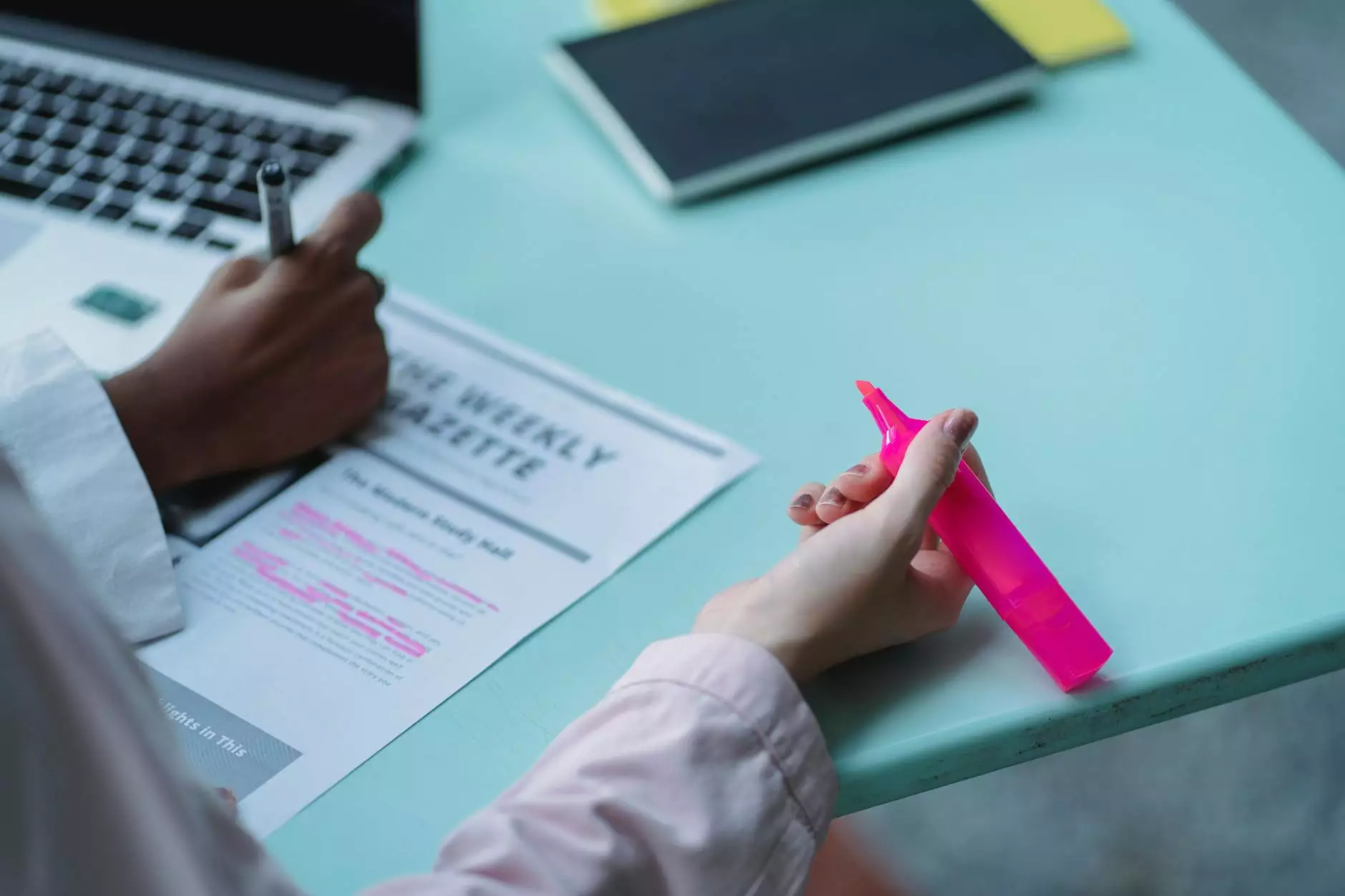
In an era where technology defines business growth, data annotation in machine learning emerges as a pivotal factor for enhancing efficiency and precision across various sectors, including Home Services and Keys & Locksmiths. As artificial intelligence (AI) continues to evolve, the need for accurate and reliable data has never been more essential. This article delves into the significance of data annotation, its impact on machine learning models, and how businesses, especially in niche markets like locksmiths, can leverage these advancements for superior service delivery.
Understanding Data Annotation
Data annotation is the process of labeling or tagging data to make it understandable for machine learning algorithms. It involves categorizing data into well-defined classes so that AI systems can learn from these examples. This practice is crucial because machine learning models require large quantities of well-annotated training data to function effectively.
The Role of Data Annotation in Machine Learning
When it comes to machine learning, the performance of algorithms heavily relies on the quality of the input data. Here’s why data annotation is so vital:
- Improved Accuracy: Accurate annotations ensure that AI systems can recognize patterns and make predictions more effectively.
- Reduced Bias: Thoughtful annotation processes help in minimizing biases in datasets, leading to more equitable AI applications.
- Enhanced Learning: Well-annotated data allows machine learning models to generalize better, resulting in improved performance across diverse scenarios.
Application in Home Services and Locksmiths
The home services industry, particularly the sector dealing with locksmiths, can significantly benefit from data annotation in machine learning. Here are several applications:
1. Smart Lock Systems
As smart lock technology advances, machine learning plays a crucial role in security systems. Data annotation helps in:
- Identifying Patterns: Annotated data can help models learn from security breaches and adapt to recognize potential risks.
- User Behavior Analysis: By understanding how users interact with locks, businesses can enhance security features tailored to user habits.
2. Location-Based Services
For locksmiths, providing services that rely on location data is essential. Here’s how data annotation aids in enhancing these services:
- Service Requests Optimization: By analyzing annotated data, companies can predict demand spikes in specific areas, deploying resources more efficiently.
- Route Optimization: Annotated maps can feed machine learning algorithms to ensure locksmiths take the fastest routes, improving response times.
3. Customer Interaction Improvements
Machine learning models trained on annotated customer interactions can significantly augment customer service:
- Chatbots and Virtual Assistants: Properly annotated conversational data can improve the understanding and responsiveness of customer service bots, making them more effective in addressing concerns.
- Feedback Implementation: Annotated feedback data can help locksmiths refine their services based on customer satisfaction metrics.
Challenges in Data Annotation
While the benefits of data annotation in machine learning are clear, there are several challenges that businesses may encounter:
1. Quality Control
Ensuring the accuracy of annotations is critical. Inaccurate labeling can lead to a cascade of errors in machine learning models, undermining their effectiveness.
2. Scalability
As businesses grow, the volume of data increases, making it challenging to maintain a consistent quality of annotation. Adopting automated annotation tools can help.
3. Cost Factor
Outsourcing data annotation can incur significant costs, which might deter small businesses. However, investing in high-quality annotation often pays off through improved machine learning outcomes.
Future Trends in Data Annotation and Machine Learning
The landscape of data annotation and machine learning is continuously evolving. Here are some expected trends:
1. Automation and AI-Assisted Annotation
Tools that use AI to assist in data annotation are on the rise, allowing organizations to speed up the process while maintaining accuracy. Automated systems can suggest labels, but human oversight remains crucial to resolve ambiguities.
2. Unsupervised Learning Techniques
As machine learning models become more sophisticated, there will be greater reliance on unsupervised learning methods that require less annotated data, broadening applications.
3. Collaborative Annotating Platforms
Platforms that facilitate collaboration among annotators will emerge, allowing businesses to leverage diverse inputs and expertise, enhancing the quality of annotations.
Conclusion
In conclusion, data annotation in machine learning is transforming the operational landscape for businesses in the home services and locksmith sectors. By ensuring that data is accurately labeled, companies can harness the power of AI to optimize operations, enhance customer satisfaction, and ultimately drive growth.
As we look to the future, embracing the prospects of automated tools, unsupervised learning, and collaborative platforms will be key to staying ahead in this digitized world. Investing in data annotation is not just a strategic advantage; it is a necessity for businesses aiming to thrive in a competitive marketplace.
Keymakr.com stands as a beacon for locksmiths and home service providers looking to integrate machine learning solutions with robust data annotation strategies. By staying informed and adaptive to the evolving technological landscape, your business can unlock the potential of data and machine learning, paving the way for success in the 21st century.
data annotation machine learning