The Ultimate Guide to Image Classification Annotation Tools
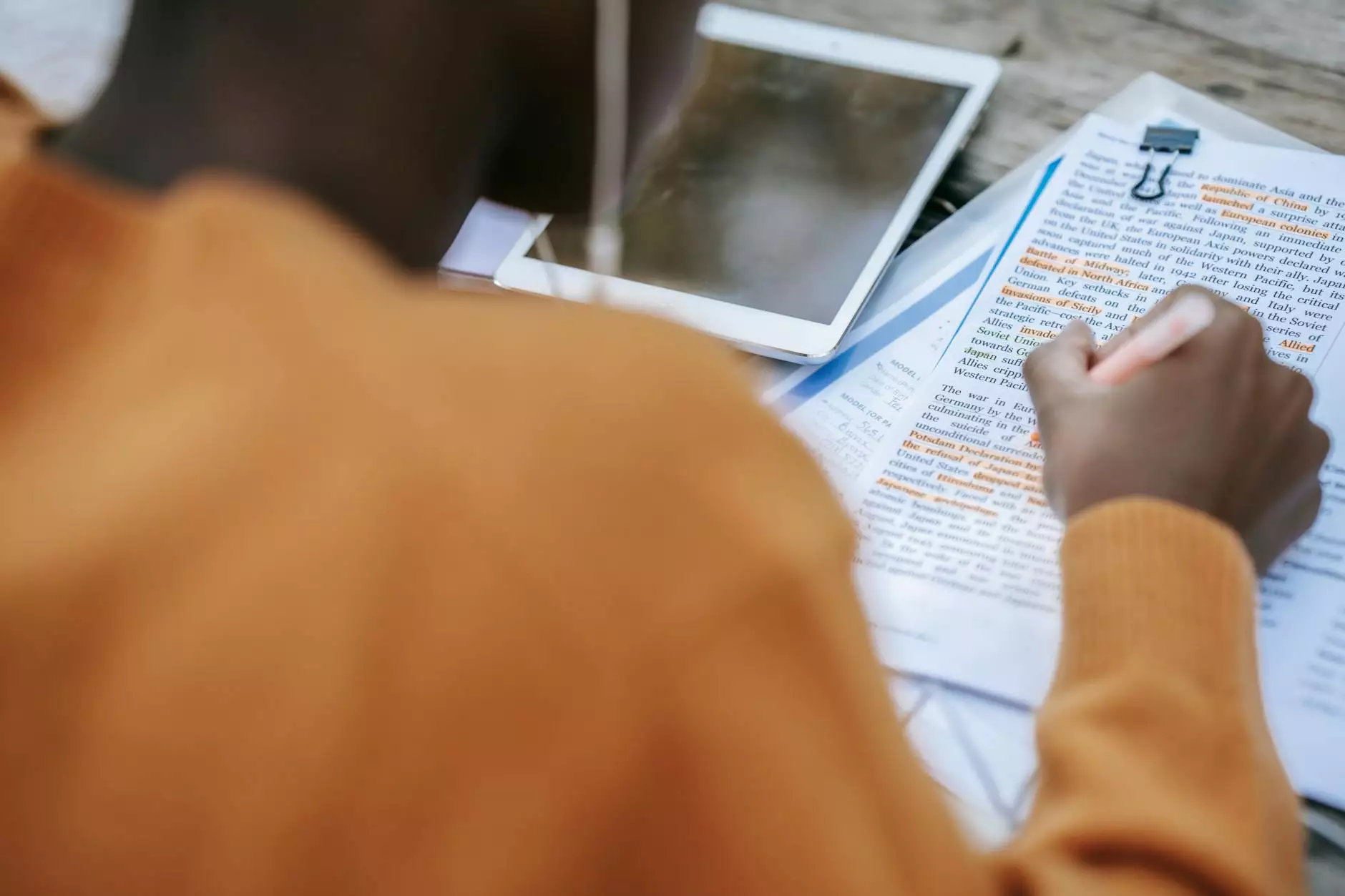
In the contemporary landscape of artificial intelligence (AI) and machine learning (ML), having high-quality data is crucial for the development of efficient algorithms. One of the essential processes in preparing data for AI models is data annotation, particularly image classification annotation. This article delves deeply into image classification annotation tools, their significance in the realm of business, and how they can transform the data annotation process into a seamless venture.
Understanding Image Classification Annotation Tools
Image classification annotation tools are software applications designed to aid in the process of tagging images with labels that signify what is depicted in them. This type of data annotation tool plays a pivotal role in training machine learning models by providing categorized image datasets.
What is Image Classification Annotation?
Image classification annotation refers to the method of assigning labels to images based on predefined categories. For example, in an image of a cat, the label would be "cat," while for a dog, it would be "dog." This process enables machine learning models to understand and categorize images accurately.
Importance of Image Classification Annotation Tools for Businesses
Businesses across various industries can significantly benefit from the implementation of image classification annotation tools. Let's explore some of the key benefits:
- Enhanced Data Accuracy: Image classification annotation tools ensure that annotations are precise and consistent, which is vital for the training of reliable AI models.
- Increased Efficiency: Automating the annotation process with advanced tools accelerates operations, leading to faster time-to-market for AI products.
- Scalability: Organizations can scale their data annotation efforts effectively as their needs grow, without compromising quality.
- Cost-Effective Solutions: By utilizing image classification annotation tools, businesses can reduce labor costs and achieve more with less.
- Improved Model Performance: High-quality annotated data leads to better-performing models, resulting in superior product offerings.
Key Features of Effective Image Classification Annotation Tools
When selecting an image classification annotation tool, certain features are essential to consider. Here are some of the most important:
User-Friendly Interface
A user-friendly interface simplifies the annotation process, allowing users with varying levels of expertise to work effectively. The easier a tool is to use, the more efficient the data annotation process becomes.
Advanced Annotation Options
Look for tools that offer various annotation methods beyond simple labeling. Some might include polygon, bounding box, or segmentation capabilities, which can be crucial for detailed projects.
Integration Capabilities
The ability to integrate with existing systems or platforms is essential. Tools that connect seamlessly with project management software can help streamline workflows.
Quality Assurance Features
Quality assurance tools that allow for validation and review of the annotations help ensure data integrity. This could include features for consensus checking and automated feedback loops.
Collaboration Tools
Annotation often requires teamwork; hence, collaboration features enable multiple users to work on the same project efficiently, facilitating communication and sharing of insights.
How to Implement Image Classification Annotation in Your Business
Implementing an image classification annotation tool in your business involves several steps:
- Define Your Objectives: Clearly outline what you aim to achieve with image classification. Identify the specific use cases and outcomes you expect.
- Select the Right Tool: Choose an image classification annotation tool that best fits your business requirements based on the features outlined previously.
- Train Your Team: Educate your team on how to use the tool effectively. This includes understanding the annotation process, quality assurance practices, and workflow management.
- Start with a Pilot Project: Begin with a small, manageable project to understand the workflow and identify any challenges.
- Iterate and Improve: Use feedback from the pilot to make improvements before scaling your annotation efforts.
Case Studies: Successful Implementations
Understanding how other businesses have successfully adopted image classification annotation tools can provide valuable insights. Here are a few examples:
Case Study 1: E-Commerce Industry
A leading e-commerce platform implemented an image classification annotation tool to enhance its product categorization system. By automating the annotation process, the company improved the accuracy of search results and customer recommendations. The result was a significant increase in user engagement and sales conversion rates.
Case Study 2: Healthcare Sector
A healthcare provider utilized image classification annotation tools to analyze medical imaging data for diagnostic purposes. Annotating images of X-rays and MRIs allowed the development of a machine learning model that accurately identified conditions such as pneumonia and fractures. This not only streamlined the diagnosis process but also improved patient outcomes.
Challenges and Solutions in Data Annotation
Despite the advantages, businesses often face several challenges while using image classification annotation tools. Here are some common hurdles and possible solutions:
Challenge 1: Resource Management
Solution: To manage resources effectively, consider leveraging a hybrid approach that combines in-house expertise with external annotation services. This allows for flexibility while keeping costs in check.
Challenge 2: Annotation Quality
Solution: Implement a robust quality control system where annotations are regularly reviewed and validated. This ensures high standards are maintained throughout the process.
Challenge 3: Keeping Up with Technology
Solution: Stay informed about advancements in data annotation technology. Regularly update your tools and methods to leverage the latest features and functionalities.
The Future of Image Classification Annotation Tools
The landscape of image classification annotation is continuously evolving. As AI and machine learning technologies advance, we can expect to see:
- Increased Automation: Future tools will feature even more sophisticated automated annotation capabilities, significantly reducing manual efforts.
- AI-Powered Quality Checks: Tools might incorporate AI algorithms to check the quality of annotations autonomously, further enhancing data reliability.
- Greater Accessibility: The rise of cloud-based annotation platforms will make these tools more accessible to a broader range of businesses, regardless of size.
Conclusion
In conclusion, employing image classification annotation tools is essential for businesses venturing into the world of AI and ML. The advantages these tools provide—enhanced data accuracy, increased efficiency, and improved model performance—are undeniable. By investing in the right tools and strategically implementing them, organizations can not only streamline their data annotation processes but also drive innovation and maintain a competitive edge in their respective industries.
As businesses strive to adapt to an ever-changing technological landscape, embracing tools that facilitate high-quality data annotation will become increasingly vital. The future of AI relies on the quality of data it learns from, and image classification annotation tools are at the forefront of this crucial endeavor.